Authors: Alex Andersson, Chris Lintott, Rob Fender, et al. (40 additional authors)
First Author’s Institution: Department of Physics, University of Oxford
Status: Accepted to MNRAS (open access)
One of the greatest challenges of 21st-century research is to develop methods that efficiently analyze the deluge of information that researchers collect. Even with the growing power of artificial intelligence (AI) and machine learning, the human ability to quickly find patterns and adapt to new types of information can’t be replicated. For that reason, more and more researchers are turning to citizen science, the use of volunteers to sift through their data and help categorize their results. Hubs for citizen science projects like the Zooniverse have been flourishing, with the Galaxy Zoo identification project receiving more than 50 million galaxy classifications in its first year alone!
In today’s paper, a new collaboration is using the power of citizen science to search for radio transients, sources of radio emission with fluxes (emission strengths) that vary over time. The majority of known radio transients are active galactic nuclei (AGN), the bright and turbulent centers of galaxies which can radiate light across the electromagnetic spectrum. But even these sources can be very diverse in their properties, and searches for radio transients often uncover unexpected sources of emission.
ThunderKAT (The Hunt for Dynamic and Explosive Radio transients with MeerKAT) is a survey project using the MeerKAT radio telescope array to actively monitor known radio transient sources and search for new ones. Using MeerKAT allows researchers to monitor sources on a roughly weekly basis, with very high sensitivity and a large field of view which allows for the serendipitous discovery of other nearby transient sources.
Finding a Nucleus in a Haystack
In the four years that ThunderKAT has been operating, they have generated over 100 TB of raw data, which must first be processed to create a wide-field image. Processing this data is hugely computationally intensive on its own, and a wide-field image can contain hundreds of sources which would need to be analyzed. Using citizen scientist volunteers, ThunderKAT can efficiently find potential transient candidates in each image and discard uninteresting sources. As shown in Figure 1, they generated the light curves (the flux of the emission over time) for each source as well as the radio image, and presented them to the volunteers. The top row shows an example of a stable (i.e. non-transient) candidate, while the bottom row is an example of a transient source.
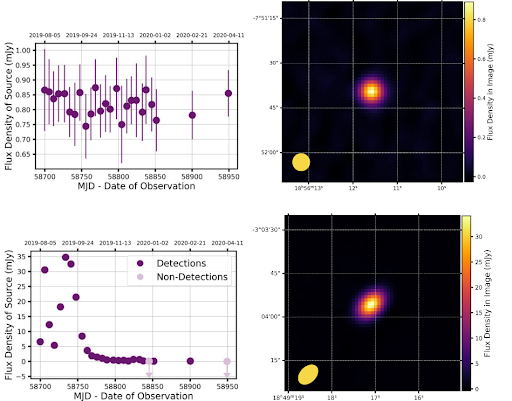
ThunderKAT drew in over 1,000 volunteers in their first three months, and classified 8,874 sources. Each source was analyzed by 10 volunteers. For a candidate to be labeled as a potential transient, at least 4 of the 10 volunteers needed to label it as a transient. Only 381 sources passed this cutoff which demonstrates the power of citizen scientists to efficiently reduce the time and processing power needed to make new discoveries. Radio astronomers visually inspected each candidate and confirmed that 168 of them are true transients, of which 142 have never been published before!
What Are These New Transients Like?
To get a better idea of the properties of each candidate, the ThunderKAT team calculated two statistical parameters: the statistical significance of the candidate (), correlated to its average flux, and its variability (V). Figure 2 is a scatter plot of all the candidates evaluated by citizen scientists, with gray points representing non-transient sources and colored points representing transient sources. Points circled in green are previously known transient sources. This plot shows that in addition to recovering the brightest (high
) known transient sources, this survey was able to discover a variety of sources at low statistical significance and low variability, demonstrating the power of citizen science!
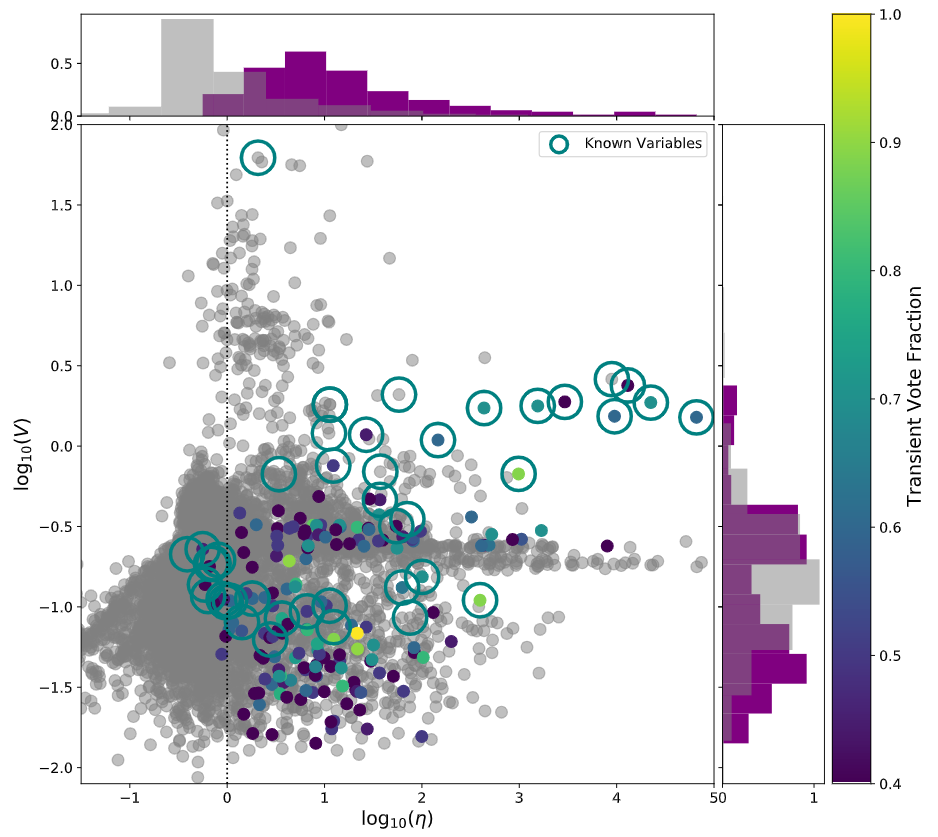
Most of the sources are AGN, powerful sources of emission thought to be powered by the central supermassive black holes inside galaxies. But there are a few unique sources in this sample as well, including an oxygen-rich evolved star creating a powerful stellar wind surrounded by a dusty atmosphere. This environment creates masers, a phenomenon where photons from the star stimulate molecules (in this case, OH molecules) to emit light at microwave frequencies.
So what’s next for the ThunderKAT team? In addition to having loads of new, interesting sources to follow up on at many different wavelengths, they’re able to study the successes and failures of this first classification project and figure out ways to get even better results next time. For instance, citizen scientists had the most accurate classifications on bright objects with smooth, long-term variations in their light curves. Conversely, objects with very few points on their light curves, or measurements with large error bars, were the source of the most inaccurate classifications. Going forward, the team can apply these findings to create better onboarding procedures and better data products for the most efficient reporting. If you’d like to lend a hand when there’s more data to analyze, you can check out the project page as well as other citizen science projects on the Zooniverse website!
Astrobite edited by William Lamb
Featured image credit: Morganoshell