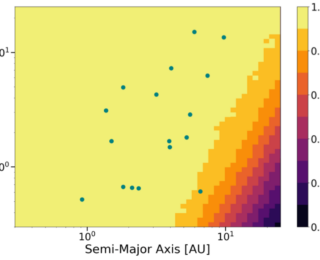
How Common are Solar Systems Like Our Own?
How common are systems like our own solar system? Do big and small planets often form together?
How common are systems like our own solar system? Do big and small planets often form together?
In today’s bite, the authors use simulations to explore turbulence a possible mechanism for the observed x-ray emissions in the coronae of black holes.
Today’s bite delves into the tragic final encounter between a supermassive black hole and its stellar neighbour that came a bit too close.
Neutron stars (NSs) are the most extreme objects known, composed of a form of matter so extraordinarily dense that it teeters on the brink of collapse into a black hole.
Everyone wants to find a habitable planet. The authors of today’s paper make a compelling case that the HR 5183 system is not the best place to look.
Pulsar timing arrays could localise individual sources of gravitational waves to host galaxies. The problem is, it’s so computationally difficult! This paper shows us a faster way.