Title: The impact of satellite trails on Hubble Space Telescope observations
Authors: Sandor Kruk, Pablo García-Martín, Marcel Popescu, Ben Aussel, Steven Dillmann, Megan E. Perks, Tamina Lund, Bruno Merín, Ross Thomson, Samet Karadag, Mark J. McCaughrean
First Author’s Institution: Max-Planck Institute for Extraterrestral Physics (MPE), Garching bei München, Germany
Status: Published in Nature Astronomy [open access]
Satellite constellations, or large clusters of satellites in our low Earth orbit (LEO; an altitude <20,000 km from Earth’s surface), streak across science images, potentially ruining observations and rendering distant astrophysical sources nearly unmeasurable. A common response to these issues raised by astronomers is to move observational equipment to LEO as well. Today’s paper covers exactly such a case, in which the Hubble Space Telescope (HST; currently in orbit 538 km above the Earth) still suffers the effects of satellite streaks. This study, the first of its kind, scans the archive of Hubble measurements from 2002–2021.
The Need
As more satellites crowd our low Earth orbit, astronomers are voicing concern about the impacts on astronomy, and whether or not it will be possible to continue to observe the sky from Earth. In this sense, today’s authors report that “observations affected by artificial satellites can become unusable for scientific research,” (italics added by Astrobite author).
This news can be disheartening, as decades of careers, budgets, and collaborations align to produce scientific instruments and observing campaigns, often on a budget. As the authors point out, redirected scientific efforts to satellite mitigation are growing to cover “costly infrastructures and mitigation efforts.”
As more satellites join LEO, a baseline for comparison becomes more valuable to enable future studies monitoring the impact of satellite megaconstellations on space-based astronomy. Today’s authors search HST archival images from 2002 to 2021 to uncover what percentage of images have been streaked and how that number changes over time.
The size of the streak in HST data varies depending on the satellites’ locations. Those in the upper portion of LEO (defined as 1,000 to 2,000 km by the authors) will be more visible to HST more often, as the camera’s field of view covers more of these orbits. Therefore, satellites in this range will appear more frequently in the data. Their great distance from the craft means their streaks are narrower, as shown in Figure 1a (top left and middle). Conversely, satellites closer to HST’s orbit, though less frequently interrupting, will have wide streaks across the data (Figure 1a, top right). Though a spread of 2,000 km may seem like a wide berth for satellites to occupy LEO, one of the main Starlink shells sits at 550km from Earth’s surface— a mere 12 km above HST. The authors calculate that the satellites will produce a streak of 120 pixels across the Advanced Camera for Surveys (ACS) onboard, impacting the science achievable with the data taken.
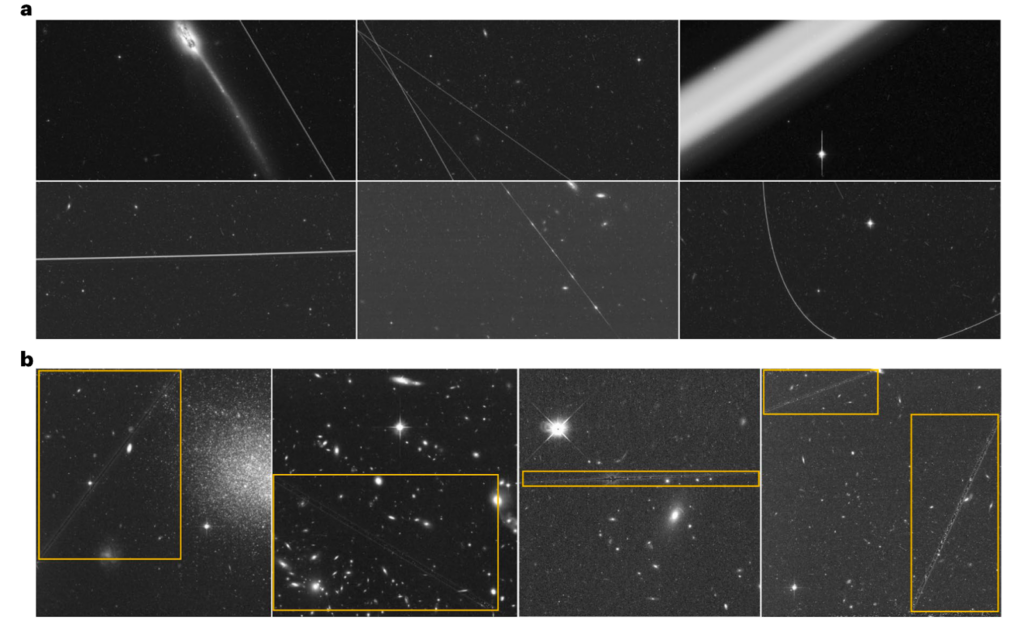
Sifting Out the Streaks
The authors further compare two different machine learning (ML) methods of satellite streak identification and two types of HST images: individual exposures (~11min) and composite (stacked) images (~35 min) available in the eHST archives.
The first ML algorithm is one that already exists in the field, DrizzlePac, historically applied to reject cosmic rays (mysterious, stray high-energy particles that occupy their very own field of study; learn more about them in this recent Bite). The ML model is a “binary classifier,” meaning it simply accepts or rejects images based on a defined criterion. While this method was good at identifying satellite streaks in archival individual exposures (of 114,607 HST images, it found 3,157 individual exposures contained satellite trails, of which only 205 cases were not correctly identified), the authors found this method was not easily amended to remove satellite trails, as larger artifacts were much larger than cosmic rays (as shown in the examples in Fig. 1b).
The second ML algorithm, the Google AutoML Vision multi-object-detection algorithm, was tested on the composite images. This method is slightly more complex than the former, as it uses a deep learning model built from a neural network search algorithm. A neural network differs from a binary yes/no classification in that it can include weights or biases. (If you’re interested in learning more about machine learning and neural networks, visit this Bite.)
To train the algorithm, the authors of today’s paper used trails identified by citizen science volunteers via the Zooniverse Hubble Asteroid Hunter. Of 149,816 HST composite image cutouts, the second method detected 7,990 satellite classifications, belonging to 4,322 composite images. Like the former method, the ML classifier was not correct 100% of the time, and so two of the authors combed through the classifications, locating and discarding 1,387 images with trails that were not caused by satellites. In the end, 3,217 satellite trails remained in the composite images. The authors conclude that both methods showed consistent results between the image types.
The Big Picture
So what does all of this mean about the state of HST science and the future of space-based astronomy? In the early days, from 2002—2005, HST ACS/WFC saw 2.8 ± 0.2% of its images tainted by satellites. Over a decade later, the same instrument saw this fraction nearly double to 4.3 ± 0.4% between 2018–2021 (Figure 2). Hubble’s Wide Field Camera 3 (WFC3/UVIS), which has a wider field of view (naturally) saw a similar increase from 1.2 ± 0.1% in 2009–2012 to 2.0 ± 0.2% in 2018–2021 (Figure 2).
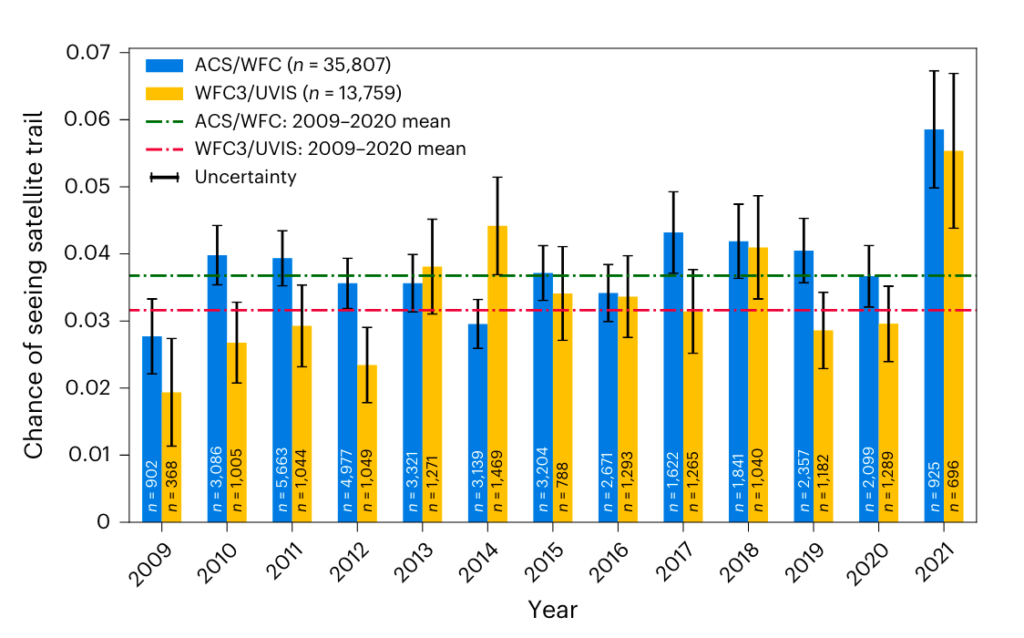
Using their newly established baseline, the authors consider the near future, in which the 1562 Starlink and 320 One Web satellites in orbit (at the time of writing) increase to 60,000–100,000 by the 2030s (only 6.5 years away!). Furthermore, they estimate a staggering 20—50% probability of a satellite crossing the HST field of view, “increasing the current fraction of affected images by an order of magnitude.” The authors remark that the 40% increase in the number of artificial satellites in orbit from 2005–2021 mirrors the 50% increase in satellite-tainted HST images. In other words, it seems that the number of satellites in orbit directly correlates to an increase in affected images taken in orbit.
Today’s paper shows existing packages like DrizzlePac could identify satellite trails, but not correct for them well. However, the authors posit more sophisticated uses of the package may be possible in the future as a cure, such as “masking out” thin streaks (identifying an anomaly and removing it) and then stacking images, not unlike science images with cosmic rays or other difficulties. However, some satellite trails are bright and wide, in which case there is no saving the image, according to the authors.
The authors further posit that deeper surveys, such as those that require long exposures and multiple measurements of the same field, are more able to discard affected exposures. Yet, other HST observing campaigns cannot afford such a luxury. For example, HST SNAP programs typically consist of only a few exposures. Conversations will no doubt continue between industry, policy, and science as the proliferation of space-based economies continues to intersect with observational astronomy.
Astrobite edited by Pranav Satheesh
Featured image credit: screencap of satellitemap.space