Title: Analysing the 21cm signal from the Epoch of Reionization with artificial neural networks
Authors: Hayato Shimabukuro and Benoit Semelin
First Author’s Institution: Sorbonne Universités, UPMC, LERMA, Observatoire de Paris, Paris, France
Status: Submitted to MNRAS, open access
Machine learning our way around the universe
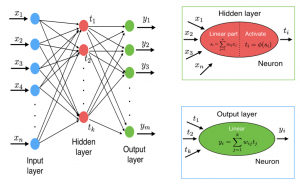
Figure 1: A neural network consists of a linear input and output layer of nodes. These nodes pass information onto hidden layers, that depending on the connections made, may further pass information forward to the output layer.
If you’re worried about the robot uprising or the much closer inevitability of machines usurping us in the workplace, then this probably isn’t the astrobite to improve your outlook on the matter. However, until the point that we have made ourselves obsolete in almost every field imaginable, we can give you some insight into how machine learning is being used to make discoveries in astronomy, cosmology, and astrophysics.
Before we can go into the cosmology of today’s bite, a bit of a primer is required on Artificial Neural Networks (ANN). ANNs are modeled after how a biological brain works, in that it takes a large number of input nodes that behave like biological neurons. These nodes receive inputs from many other nodes and are able to make, remove, or reinforce connections. In the case of ANNs, nodes are layered as seen in Fig. 1. The input layer takes information that passes to a hidden layer (which can be many hidden layers). The hidden layer has what is known as an activation function which is normally nonlinear, while the input and output layers take information in linearly.
An ANN that only speaks from input to output is known as forward-propagating, whereas in back-propagating ANNs’ forward layers can communicate with a previous layer. By making these many connections between layers of nodes, solutions to complex problems can be learned by ANNs. Training the ANNs involves feeding it data in such a way that the results you hope to achieve are easily distinguishable from those that you do not want. This is similar to how the human brain works, you give it an easily understood pattern, and the brain can extrapolate to more complex situations like those involving noise. But just like the human brain, ANNs fall into the trap of making incorrect associations.
Reionization, aka how hydrogen lost his electron friend…again
Recombination is the point in the Universe’s history where protons combine with free electrons to form neutral hydrogen. Following this epoch of recombination, galaxies and stars had yet to exist to emit light, and this period is referred to as the Dark Ages. Eventually large scale structure formation began, meaning the first stars and galaxies started to form. The ionizing radiation produced by these first structures ionized the intergalactic medium (IGM) which mostly consisted of neutral hydrogen and this is known as the Epoch of Reionization (EoR).
This epoch is cloaked in mystery, but luckily we have a way to detect and measure it, by looking at the neutral hydrogen radio emission at 21cm. This 21cm radio emission should be detectable within the next decade or so with the newest and largest radio telescope arrays, such as the Hydrogen Epoch of Reionization Array (HERA) or the Square Kilometer Array (SKA). Until we can make an accurate detection, we must rely on simulations and clever analyses to understand this mysterious epoch.
Learning the EoR Parameters
The EoR has several parameters that help define how reionization progressed. In today’s bite we are only concerned with the ionizing efficiency ζ , virial temperature , and the mean free path of ionizing photons
. All of these parameters can help define how quickly and efficiently reionization occurred and the conditions surrounding it. To teach the ANNs how to estimate these parameters, the authors used 70 training datasets obtained from simulating a region of neutral hydrogen as it was ionized.
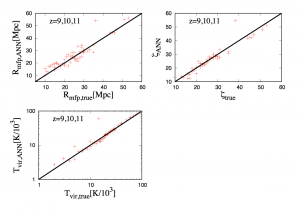
Figure 2: The estimated parameters by the ANN show that with enough training they can accurately recover the simulation initial parameters.
The ANN inputs were the 21cm measurements from the simulation, and the outputs were the measurements of and
. In Fig. 2, the results of training the back-propagating ANN show that with minimal training datasets it is able to recover the initial simulation parameters with amazing clarity. To further show the validity of using ANNs to estimate parameters, after training the ANN on ideal 21cm measurements the authors tested it with good results on different simulated data with noise added. The results of all the ANN parameter estimation trials showed that it had difficulty in recovering the mean free path,
. This is somewhat expected as
is a less sensitive parameter at high redshifts (really far back in time) and only becomes easier to measure at low redshift where reionization is in its late stages. This is the case because
grows larger as more and more neutral hydrogen becomes ionized.
The authors clearly demonstrate that ANNs have a place in parameter estimation, and that their ability to recognize patterns in data is astounding. However we aren’t quite at the level of the machine cosmologist, so I’m not too convinced that the machines will be taking our jobs in astronomy and cosmology anytime soon.
Thank you for introducing our paper!