Authors: Jade Powell, Alberto Iess, Miquel Llorens-Monteagudo, Martin Obergaulinger, Bernhard Müller, Alejandro Torres-Forné, Elena Cuoco, José A. Font
First Author’s Institution: Centre for Astrophysics and Supercomputing, Swinburne University of Technology, Hawthorn, VIC 3122, Australia
Status: Accepted for publication in Physical Review D [open access]
Have you ever seen a gravitational wave signal? It looks something like this:
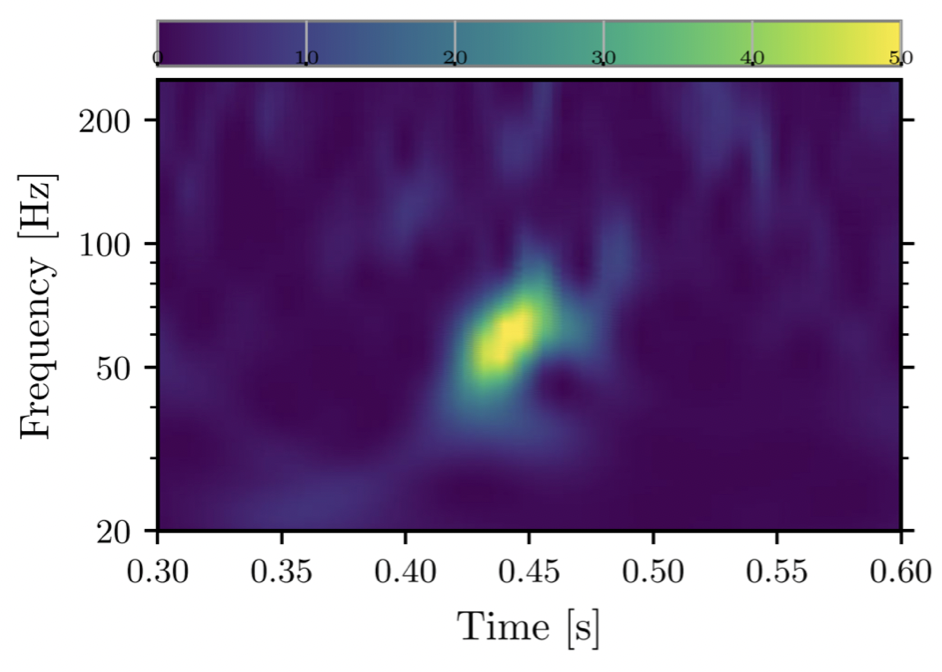
What if I told you I could look at this signal in Figure 1 and tell you it was caused by two merging black holes? What if I go a step further and also tell you that the black holes weigh 85 and 66 times the mass of our sun?
This might seem like a case of some fantastic fortune-telling, but only it’s not! When such highly energetic phenomena occur in the universe, they cause huge ripples in the fabric of spacetime, creating gravitational waves. Astronomers can model what kind of gravitational waves can be formed from different events (such as merging black holes or neutron stars) and can predict what the signal will look like! When we see a gravitational wave signal from the gravitational wave detectors on Earth, we can have a pretty good idea of what astronomical event caused that particular signal.
One such event is a core-collapse supernova. This is one of the ways by which a star with a high mass ends its life. The formation of an iron core abruptly halts the fusion process in the star. It leads to the launching of a shock wave from the core, which can be characterized as an explosion. But what powers this explosion? One possible way is the neutrino-driven explosion mechanism, where the explosion is powered by runaway small particles called neutrinos. The other mechanism is when rotating magnetic fields power the explosion. Luckily for us, imprints of these mechanisms can be found in the gravitational wave signals they produce.
The authors of today’s paper predict how the wave looks when a supernova collapses by several possible mechanisms. They then use computing techniques to see if they can accurately predict which mechanism caused the signal, just based on the detected signal. Current gravitational wave detectors cannot detect signals from supernovae that collapse (they primarily detect mergers between black holes and neutron stars), but the next-generation gravitational wave detectors should be sensitive enough to pick up such signals. Also, when the next generation of detectors comes along, our world will likely have advanced in machine learning and signal processing techniques. So, it is only appropriate to determine which machine learning technique can accurately determine what caused the gravitational wave signal, which is what the authors of this paper set out to do! Much like the Bene Gesserit, their plans are measured in centuries (more like decades, but you get the reference!)
Unveiling these plans
The waveforms are modeled based on four scenarios (Figure 2):
- The core collapses without an explosion and quickly forms a black hole, leading to low-frequency gravitational waves.
- The core collapses and explodes via neutrinos, leading to specific characteristics of the waveforms that result in high frequencies (above 500 hertz).
- The core collapses, and the explosion is powered by the rotation of the core and the associated magnetic fields, leading to high amplitudes of the resulting gravitational wave.
- A fourth chirplet model was chosen to model a signal that does not belong to one of the above criteria (but could replicate an actual gravitational wave signal, perhaps from some other source) to see what the machine learning techniques do if it encounters such a signal.
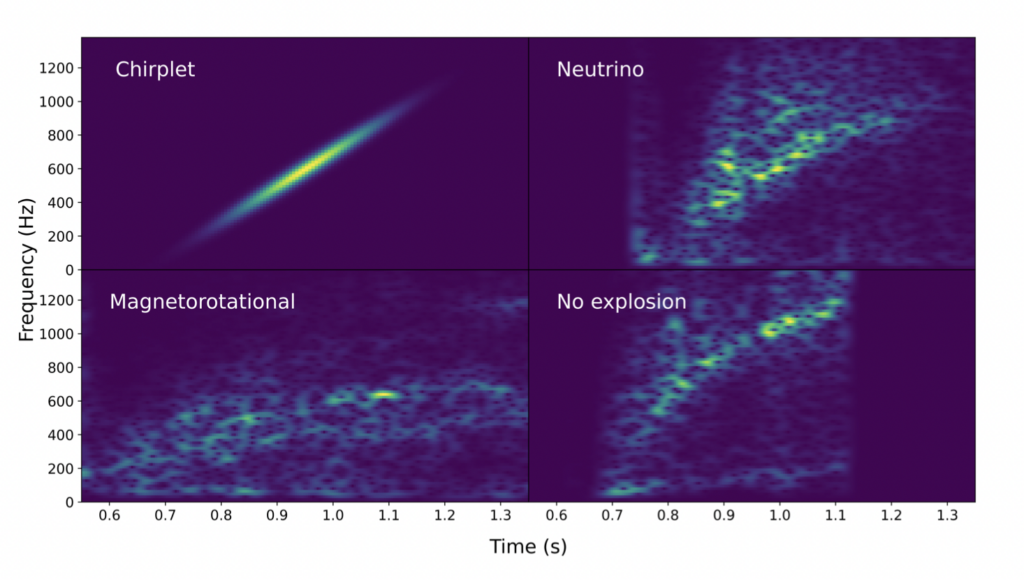
The three machine learning techniques the authors explore are:
- Principal Component Analysis (PCA) and Bayesian Model Selection: With this technique, you can simplify your data and find important patterns in the information gathered from gravitational wave detectors.
- Dictionary learning: Complex gravitational wave signals are broken down into simpler components and then analyzed.
- 2D Convolution Neural Networks: These networks apply filters to extract features from images of the gravitational wave signals, downsample for efficiency, and improve predictions through learning.
Since gravitational wave signals are usually faint and often get lost in the noise produced by the various detectors, the authors also model the noise levels theorized to be measured by each of the detectors and bury the actual signal in them. The modeled waveforms are then analyzed using the techniques mentioned above for three different next-generation detectors, the advanced Laser Interferometer Gravitational-Wave Observatory (LIGO), the Einstein Telescope (ET), and the Neutron Star Extreme Matter Observatory (NEMO).
Do we have one technique that can determine the origins accurately? (aka the Kwisatz Haderach!)
Overall, the authors find that all techniques in all the detectors accurately classify the chirplet signal as an outlier. The accuracy of the rest of the classification mechanisms varies depending on the detector, but overall, the PCA+Bayesian modeling predicts the origins more accurately than the other two techniques across all the detectors. While the distinction between the non-exploding and exploding cases is well classified, there still appears to be some ambiguity while accurately pinpointing the origin of the two exploding signals between the neutrino-driven and magnetorotational mechanisms.
Maybe, but there are other prospects!
This paper shows promise that it is possible to predict the astrophysical origins of core collapse supernovae in the universe. With improvements in detector capabilities and computational methods, predictability is expected to improve in the upcoming years. The future is truly an exciting time to understand the mysteries of the universe from the comfort of the control rooms of the gravitational detectors on Earth!
Astrobite edited by Lucie Rowland
Featured image credit: ESO/L. Calçada